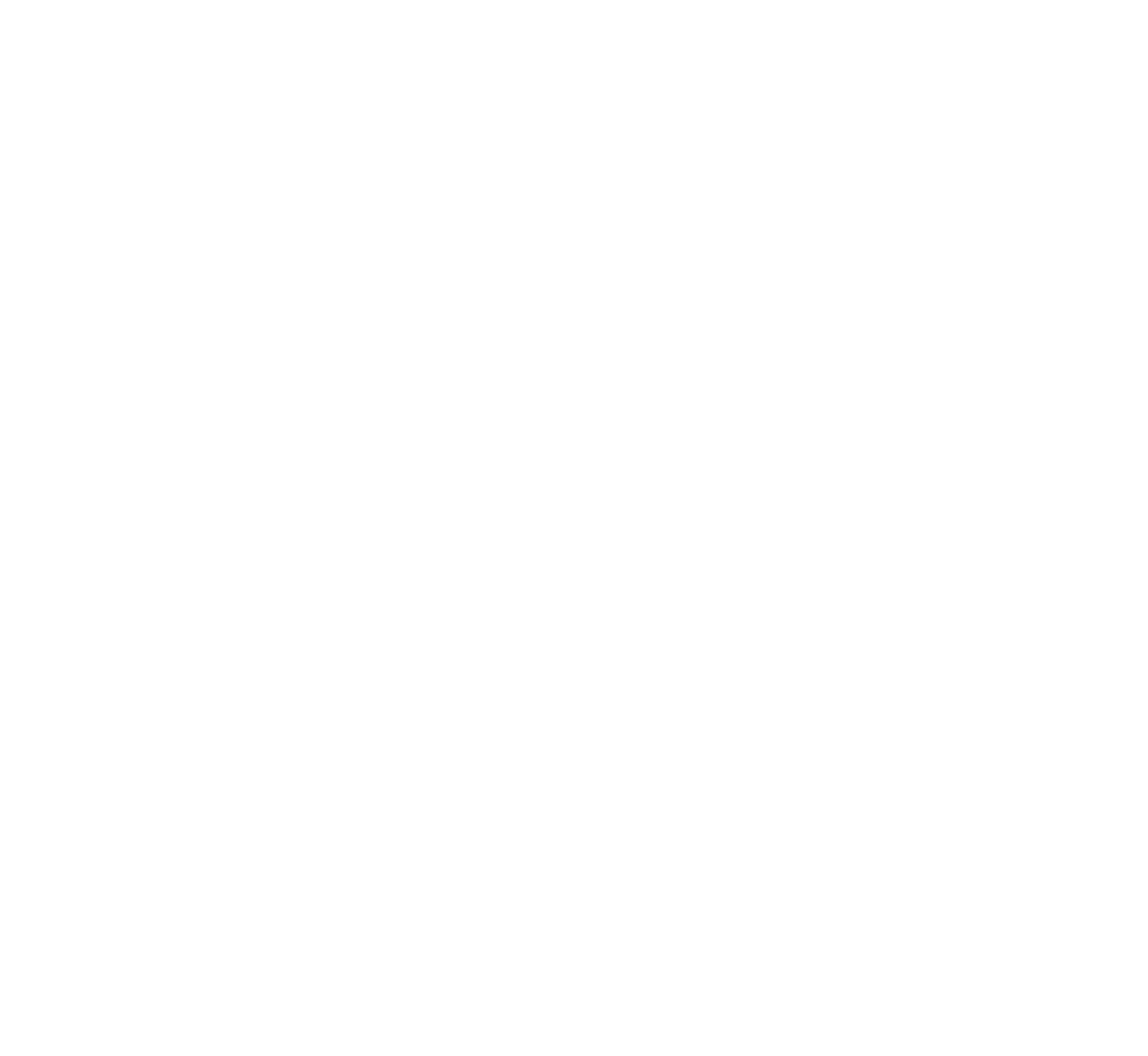
Virtual Screening of Potent Inhibitors for Mycobacterium tuberculosis Arabinogalactan Synthesis
Main Article Content
Abstract
Background: Tuberculosis remains the most prevalent infectious disease, requiring the identification of new targets and drugs due to emerging drug resistance for Mycobacterium tuberculosis. Virtual screening has the potential to be highly valuable for identifying new drug candidates by searching through compound databases. The present study involved screening a library of around 3.5 million selected compounds from the ZINC20 database and a reference inhibitor library from literature to identify strong inhibitors against the Decaprenylphosphoryl-β-D-ribose 2'-epimerase (DprE1) enzyme of M. tuberculosis through virtual methods.
Methods: A two-step selection of compounds was carried out from the ZINC20 database using stringent reference physicochemical ranks based on the reference inhibitor library. The resulting library comprised 2700 compounds from the ZINC database along with 21 reference inhibitors from the literature exhibiting MICs of 10 μM or lower. Docking simulations were performed for a total of 2721 compounds based on the AutoDock Vina tool using python-based computational drug discovery software, PyRx against the crystal structure of M. tuberculosis DprE1 enzyme (Protein Data Bank, PDB: 4P8K).
Results: After docking analysis of the selected final library (2721 in total), we determined 21 molecules as top-ranked compounds. The top-ranked compounds from the ZINC20 library had a ΔG in the range of -11 and -11.9 compared to -7.7 and -10.9 of the selected 21 reference inhibitors with MICs of 10 μM or lower. Among the whole library, ZINC000009429240 and Ref3 emerged as the highest ranked compounds, exhibiting a ΔG value of -11.9 and -10.9, respectively. ZINC000009429240 and Ref3 were determined to show H-bond interactions with 228S and 418K versus 132H, 228S and 60Y, respectively.
Conclusion: Here, we virtually identified a largely distinct set of compounds as effective inhibitors for the DprE1 enzyme of M. tuberculosis. The data gathered in this study may have the potential to be developed into effective drug molecules.
Cite this article as: Yaşar N, Ayvaz AS, Dilek G. Virtual screening of potent inhibitors for Mycobacterium tuberculosis arabinogalactan synthesis. Trends Pharm. 2024, 1, 9, doi: 10.5152/TrendsPharm.2024.23009
Article Details
References
1. Salina EG. Mycobacterium tuberculosis infection: Control and treatment. Microorganisms. 2023 Apr 18;11(4):1057. [Crossref]
2. Chakaya J, Khan M, Ntoumi F, et al. Global Tuberculosis Report 2020 - Reflections on the global TB burden, treatment and prevention efforts. Int J Infect Dis. 2021;113 Suppl 1(Suppl 1):7-12. [Crossref]
3. Zumla A, Nahid P, Cole ST. Advances in the development of new tuberculosis drugs and treatment regimens. Nat Rev Drug Discov. 2013 May;12(5):388-404. [Crossref]
4. Singh V, Chibale K. Strategies to combat multi-drug resistance in tuberculosis. Acc Chem Res. 2021 May 18;54(10):2361-2376. [Crossref]
5. Oh S, Trifonov L, Yadav VD, et al. Tuberculosis drug discovery: a decade of hit assessment for defined targets. Front Cell Infect Microbiol. 2021;11:611304. [Crossref]
6. Abrahams KA, Batt SM, Gurcha SS, et al. Dpre2 is a molecular target of the anti-tubercular nitroimidazole compounds pretomanid and delamanid. Nat Commun. 2023;14(1):3828. [Crossref]
7. Makarov V, Neres J, Hartkoorn RC, et al. The 8-pyrrole-benzothiazinones are noncovalent inhibitors of Dpre1 from Mycobacterium tuberculosis. Antimicrob Agents Chemother. 2015;59(8):4446-4452. [Crossref]
8. Tiwari R, Miller PA, Chiarelli LR, et al. Design, syntheses, and anti-TB activity of 1,3-benzothiazinone azide and click chemistry products inspired by BTZ043. ACS Med Chem Lett. 2016;7(3):266-270. [Crossref]
9. Chikhale R, Menghani S, Babu R, et al. Development of selective Dpre1 inhibitors: Design, synthesis, crystal structure and antitubercular activity of benzothiazolylpyrimidine-5-carboxamides. Eur J Med Chem. 2015;96:30-46. [Crossref]
10. Naidu A, Nayak SS, Lulu SS, et al. Advances in computational frameworks in the fight against TB: The way forward. Front Pharmacol. 2023;14:1152915. [Crossref]
11. Irwin JJ, Tang KG, Young J, et al. ZINC20-A free ultralarge-scale chemical database for ligand discovery. J Chem Inf Model. 2020;60(12):6065-6073. [Crossref]
12. Amado PSM, Woodley C, Cristiano MLS, et al. Recent advances of Dpre1 inhibitors against Mycobacterium tuberculosis: Computational analysis of physicochemical and ADMET properties. ACS Omega. 2022;7(45):40659-40681. [Crossref]
13. Sander T, Freyss J, von Korff M, Rufener C. DataWarrior: An open-source program for chemistry-aware data visualization and analysis. J Chem Inf Model. 2015;55(2):460-473. [Crossref]
14. Benet LZ, Hosey CM, Ursu O, et al. BDDCS, The rule of 5 and drugability. Adv Drug Deliv Rev. 2016 Jun 1;101:89-98. [Crossref]
15. Trott O, Olson AJ. Autodock Vina: Improving the speed and accuracy of docking with a new scoring function, efficient optimization, and multithreading. J Comput Chem. 2010;31(2):455-461. [Crossref]
16. Dallakyan S, Olson AJ. Small-molecule library screening by docking with PyRx. Methods Mol Biol. 2015;1263:243-250. [Crossref]
17. Neres J, Hartkoorn RC, Chiarelli LR, et al. 2-carboxyquinoxalines kill Mycobacterium tuberculosis through noncovalent inhibition of Dpre1. ACS Chem Biol. 2015;10(3):705-714. [Crossref]
18. Schrödinger L, DeLano W. PyMOL. 2020. Retrieved from http://www.pymol.org/pymol
19. Niranjan K, Srivastava R, Prakash A, et al. Virtual screening and free energy estimation for identifying Mycobacterium tuberculosis flavoenzyme Dpre1 inhibitors. J Mol Graph Model. 2021;102:107770. [Crossref]
20. Collins AP, Abdelfattah W. Structure-based virtual screening of essential Mycobacterium tuberculosis enzymes AspS and KatG for potential inhibitors. Bioinformation. 2021;17(1):101-108. [Crossref]
21. Thongdee P, Hanwarinroj C, Pakamwong B, et al. Virtual screening to identify novel potential inhibitors for glutamine synthetase of Mycobacterium tuberculosis PknB with antibacterial activity. J Chem Inf Model. 2022;62(24):6508-6518. [Crossref]
22. Kumari M, Subbarao N. Virtual screening to identify novel potential inhibitors for glutamine synthetase of Mycobacterium tuberculosis. J Biomol Struct Dyn. 2020;38(17):5062-5080. [Crossref]
23. Andrianov AM, Furs KV, Gonchar AV, et al. Virtual screening and identification of promising therapeutic compounds against drug-resistant Mycobacterium tuberculosis Β-ketoacyl-acyl carrier protein synthase I (KasA). J Biomol Struct Dyn. 2023:1-13. [Crossref]
24. Sundararajan S, Karunakaran K, Muniyan R. Structure-based virtual screening and discovery of novel inhibitors against FabD protein of Mycobacterium tuberculosis. J Biomol Struct Dyn. 2023:1-12. [Crossref]
25. Singh S, Khare G, Bahal RK, et al. Identification of Mycobacterium tuberculosis BioA inhibitors by using structure-based virtual screening. Drug Des Devel Ther. 2018;12:1065-1079. [Crossref]
26. Zhang G, Guo S, Cui H, et al. Virtual screening of small molecular inhibitors against DprE1. Molecules. 2018;23(3):524. [Crossref]
27. Anand R. Identification of potential antituberculosis drugs through docking and virtual screening. Interdiscip Sci. 2018;10(2):419-429. [Crossref]